Since the first push when we deployed data.open.ac.uk, the area of linked data for education, especially in universities, as been slowly but steadily growing. This is obviously a rather good news as a critical benefit of linked data in education (some would say, the only one worth considering) is that it creates a common, public information space for education that goes outside the boundaries of specific institutions. However, this will only happen if a certain level of convergence is happening so that shared vocabularies and schema elements are commonly used that make it possible to aggregate and jointly query data provided by different parties. Here, we try to get an overview of the current landscape in existing linked datasets in the education sector, to see how much of this convergence is happening, what are the areas of clear agreement, and the ones where more efforts might be required.
The Datasets
To look at the current state of linked data in education, we considered 8 different datasets, some provided by universities and some by specific projects. We looked at datasets that were explicitly dedicated to education (as opposed to the ones containing information that could be used for educational purposes, such as library and museum data, and the ones that have connection with education but focus on other aspects, such as the datasets from purely research institutions). Also, we view datasets in a very coarse-grained way, for example considering the whole of data.open.ac.uk as one dataset, rather than each of its sub-datasets separately. Finally, we could only process datasets with a functioning SPARQL endpoint working properly with common SPARQL clients (in our case ARC2).
From Universities:
- data.open.ac.uk which SPARQL endpoint is available at http://data.open.ac.uk/sparql
- data.bris from the University of Bristol. SPARQL endpoint: http://resrev.ilrt.bris.ac.uk/data-server-workshop/sparql
- University of Southampton Open Data. SPARQL endpoint: http://sparql.data.southampton.ac.uk/
- LODUM from the University of Muenster, Germany. SPARQL endpoint: http://data.uni-muenster.de/sparql
Others should be included eventually, but we could not access them at the time
From projects and broader institutions
- mEducator, a european project aggregating learning resources: SPARQL Endpoint: http://meducator.open.ac.uk/resourcesrestapi/rest/meducator/sparql
- OrganicEduNet a european project that aggregated learning resources from LOM repositories (see this post). SPARQL endpoint: http://knowone.csc.kth.se/sparql/ariadne-big
- LinkedUniversities Video Dataset which aggregates video resources from various repositories (see this paper). SPARQL Endpoint: http://smartproducts1.kmi.open.ac.uk:8080/openrdf-sesame/repositories/linkeduniversities
- Data.gov.uk Education which aggregates information about schools in the UK. SPARQL endpoint: http://services.data.gov.uk/education/sparql
Common Vocabularies

As everybody will always say: the important thing is the reuse shared and common vocabularies! As they are talking about similar things, it is expected that education-related datasets would share vocabularies, and that their overlaps would allow to achieve joint reuse of the exposed data. The chart above shows the namespaces that are used by more than one of the considered datasets.
Unsurprisingly, FOAF is almost omnipresent. One of the reasons for this is that FOAF is the unquestioned common vocabulary to represent information about people, and it is quite rare that an education-related dataset would not need to represent information about people. It is also the case that FOAF includes high-level classes that are also very common, especially in this sort of datasets, namely Document and Organisation.
In clear second place come vocabularies to represent information about bibliographic resources, and other published artifacts: Dublin Core and BIBO. Dublin Core is actually the de-facto standard for metadata for just about anything that can be published. BIBO, the bibliographic ontology, is more specialised (and actually rely on both Dublin Core and FOAF) to represents in particular academic publications.
Other vocabularies used include generic “representation languages” such as RDF, RDFS, OWL and SKOS (often used to represent topics), as well as specific vocabularies related to the description of multimedia resources, events and places (including building, addresses and geo-location).
Common Classes

At a more granular level, it is interesting to look at the types of entities that can be found in the considered datasets. The chart above shows the classes that are used by at least 2 datasets. This confirms in particular the strong focus on people and bibliographic/learning resources (Article, Book, Document, Thesis, Podcast, Recording, Image, Patent, Report, Slideshow).
In second place come information about educational institutions as organisations and physical places (Organization, Institution, Building, Address, VCard).
Besides generic, language-level classes other areas such as events, courses, vacancies, etc. tend to be only considered by a very small number of datasets.
Common Properties
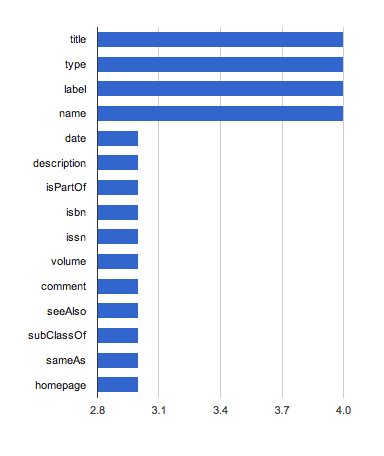
Finally, going a step further in granularity, we look through the chart above at the way common types of entities are represented. This chart show the properties used by more than 3 datasets. Once again, besides generic properties, the focus on people (name) and media/bibliographic resources (title, date, subject) is obvious, especially with properties connecting the 2 (contributor, homepage).
The representation of institutions as physically located places is also clearly reflected here (lat, long, postal-code, street-address, adr).
Doing More with the Collected Data
Of course, the considered datasets only represent a small sample, and ideally, we could draw some more definitive conclusions as the number of education-related datasets grows and are included. Indeed, in order to realise the analysis in this post, we created a script that generates VOID-based descriptions of the datasets. The created descriptions are available on a public SPARQL endpoint which will be extended as we find more datasets to include. Please let us know if there are datasets you would like to see taken into account. The charts above are dynamically generated out of SPARQL query to the aforementioned SPARQL endpoint.
Also, we will look at reflecting the elements discussed here on the vocabulary page of LinkedUniversities.org. The nice thing about having a SPARQL endpoint for the collected data is that it will make it easy to create a simple tool to explore the “Vocabulary Space” of educational datasets. This might appear useful as well as a way provide federated querying services for common types of entities (see this recent paper about using VOID for doing that), which might end-up being a useful feature for the recently launched data.ac.uk initiative (?) Another interesting thing to do would be to apply a tiny bit of data-mining to check for example what elements tend to appear together, and see if there are common patterns in the use of some vocabularies.